Unlocking the Power of Machine Learning Data Labeling
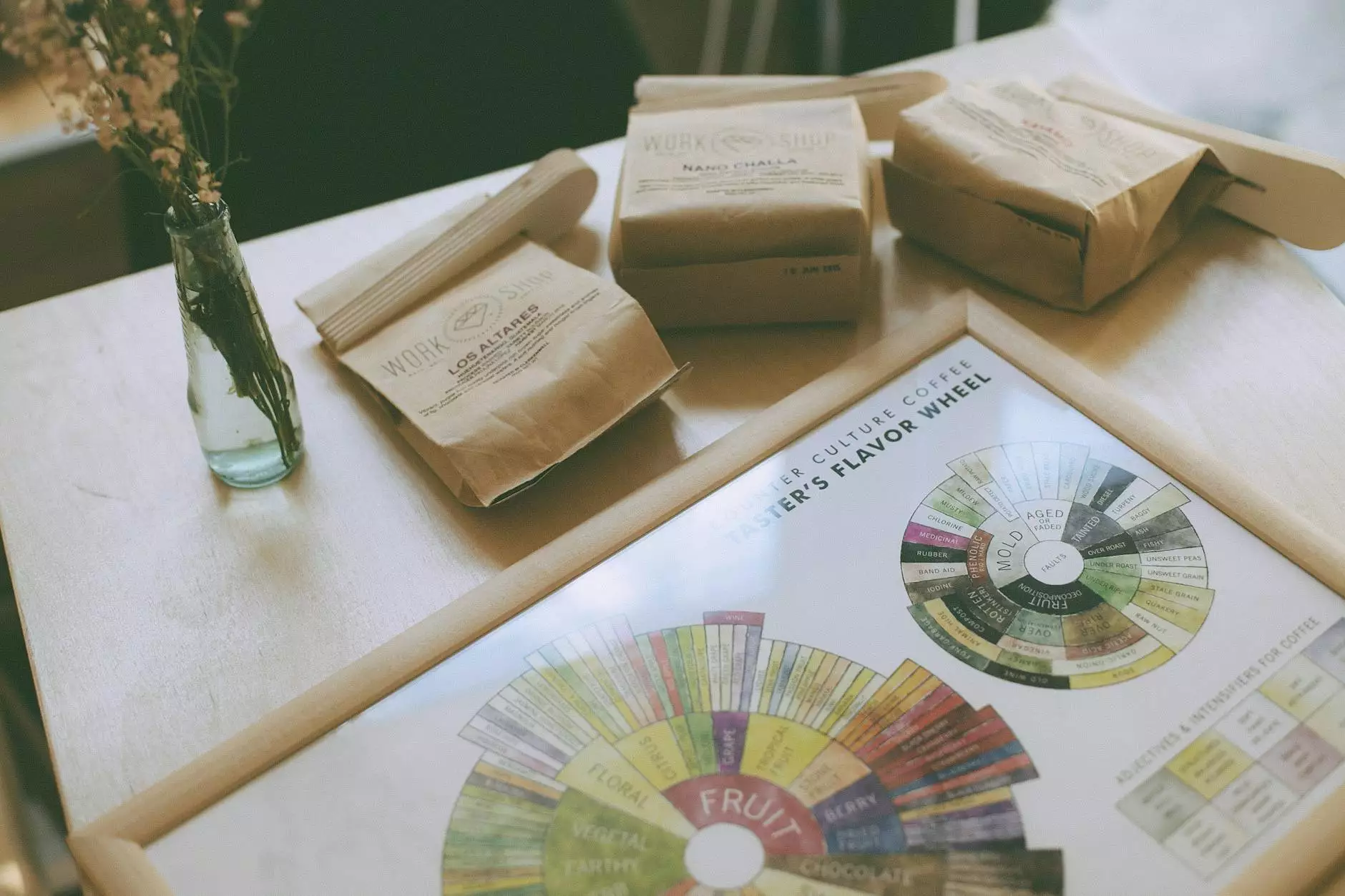
In the rapidly evolving landscape of artificial intelligence (AI), one term that continues to buzz across industries is machine learning data labeling. As the foundation upon which AI models are built, effective data labeling is paramount for the development of accurate and efficient machine learning systems. This article delves deep into the essence of data labeling, the tools and platforms available, and how businesses can tap into these resources for phenomenal growth and innovation.
Understanding Machine Learning Data Labeling
Machine learning data labeling refers to the process of annotating data with informative labels, so that machine learning algorithms can learn from it. This is akin to teaching a child to recognize objects by pointing them out and naming them. For AI systems to understand and predict accurately, they need data that is clean, organized, and appropriately tagged.
The Importance of Data Labeling in AI
Data is the new oil, and just like oil, it needs to be refined and processed to deliver value. Here are some pivotal reasons why data labeling is critical:
- Enhances Model Accuracy: High-quality labeled datasets result in better-performing models, reducing error rates significantly.
- Facilitates Supervised Learning: It allows algorithms to learn from labeled examples, making predictions on unseen data more reliable.
- Scalability: As organizations grow, they accumulate vast amounts of data. Proper labeling ensures that this data can be efficiently utilized in processing and analysis.
Types of Data That Require Labeling
Not all data is created equal, and different types of data come with distinct labeling requirements. Below are major categories of data requiring attention:
1. Images
Image labeling is critical for computer vision tasks. Examples include:
- Object Detection: Identifying multiple objects within an image and labeling them accordingly.
- Image Segmentation: Classifying each pixel in an image to understand and delineate different elements accurately.
2. Text
Natural Language Processing (NLP) relies heavily on text labeling. Activities include:
- Sentiment Analysis: Classifying text data based on sentiment (positive, negative, neutral).
- Named Entity Recognition: Identifying and categorizing key entities in text (such as names of places, organizations, etc.).
3. Videos
Video data labeling is increasingly important for applications like:
- Action Recognition: Labeling frames in a video to recognize and categorize actions.
- Object Tracking: Tracking objects across frames to improve identification accuracy.
Exploring Data Annotation Tools and Platforms
In order to effectively perform machine learning data labeling, organizations need robust tools and platforms. Here's a closer look at some popular options available:
1. Keylabs.ai: A Premier Data Annotation Platform
Keylabs.ai stands at the forefront as a premier data annotation platform. With its user-centric design, it allows for smooth collaboration between data teams. Key features include:
- Customizable Workflows: Tailor the platform to your specific business needs, optimizing the annotation process.
- Multi-format Support: Ability to handle various data types including images, text, and videos.
- Advanced Tools: Incorporate AI assistance to augment human efforts, speeding up the labeling process.
2. Amazon SageMaker Ground Truth
This is a fully managed service provided by Amazon Web Services (AWS) that enables organizations to build high-quality training datasets for machine learning. Key features include:
- Built-in Workflows: Pre-defined labeling workflows simplify the setup process.
- Human Review: Integrates human annotators to ensure the accuracy of the labeled data.
3. Labelbox
Labelbox provides a flexible and collaborative platform. Key attributes include:
- Collaboration Features: Enables team collaboration and feedback directly on the platform to streamline the labeling process.
- Real-time Progress Tracking: Allows users to view the status of ongoing annotation tasks.
Best Practices for Machine Learning Data Labeling
To ensure high-quality results, businesses should follow best practices during the data labeling process. Here are some essential strategies:
1. Define Clear Labeling Guidelines
Establish comprehensive guidelines that outline how data should be labeled. This reduces ambiguity and ensures consistency across the dataset.
2. Use a Diverse Dataset
Utilizing a diverse set of data that reflects different scenarios helps the AI model generalize better and improve its performance on unseen data.
3. Regular Quality Checks
Implement regular quality assurance checks and inter-annotator agreements to maintain the integrity of the labeled data.
4. Leverage Automation
Utilize AI-assisted tools to reduce manual labor and increase efficiency, while keeping human in the loop for verification when necessary.
The Future of Machine Learning Data Labeling
As we move towards an even more digitized world, the future of machine learning data labeling holds exciting possibilities. Here are some trends to watch:
- Increased Use of Synthetic Data: Synthetic datasets will gain traction as businesses look to augment real datasets, saving time and resources in the labeling process.
- Integration of AI with Human Labeling: A hybrid approach that leverages the strengths of both AI and human annotators will become standard, ensuring high-quality outcomes.
- Focus on Data Privacy: As data regulations become stringent, platforms will have to ensure that the data labeling processes are compliant with privacy standards.
Conclusion
Machine learning data labeling is not just a necessary task but a foundational element in the roadmap for innovative, AI-driven solutions. By adopting robust tools like Keylabs.ai and adhering to best practices, organizations can harness the true potential of their data assets. As this sector continues to grow, staying updated with the latest trends and technologies will be essential for businesses seeking to lead in their respective fields.
In conclusion, investing in high-quality data labeling processes will pave the way for improved machine learning outcomes, and ultimately, business success. As the AI landscape expands, those who prioritize excellent data practices today will be the leaders of tomorrow.