Unlocking the Power of Image Datasets for Classification
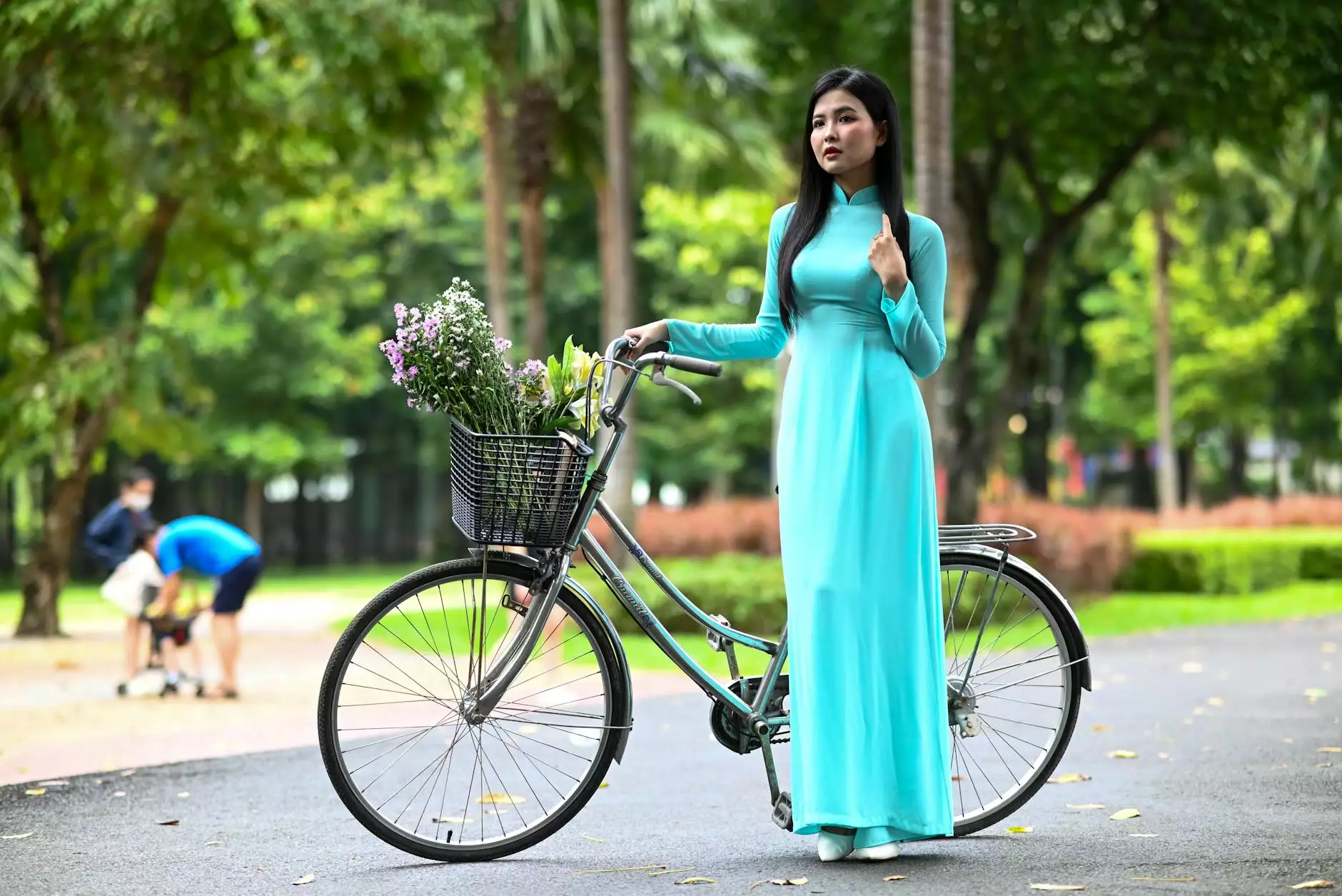
Understanding Image Datasets for Classification
The realm of machine learning and artificial intelligence has witnessed phenomenal growth, primarily due to the surge in the availability of data. Among various types of data, image datasets for classification play a pivotal role in training algorithms to recognize patterns, objects, and attributes in images. This article delves into the significance, creation, and utilization of these datasets, alongside exploring leading data annotation tools and platforms.
What are Image Datasets?
An image dataset is a collection of images that are used for various applications, including training machine learning models. These datasets contain a multitude of images, often accompanied by labels that denote the objects or features present within the images. For a dataset to be effective for classification tasks, it must be well-categorized and contain diverse representations of each class.
The Importance of Data Annotation
Data annotation is the process of labeling data to make it understandable for machine learning models. When it comes to image datasets for classification, annotating images accurately is essential. Here are some reasons why data annotation is important:
- Improved Model Accuracy: Well-annotated datasets lead to higher classification accuracy in machine learning models.
- Better Generalization: Diverse and comprehensive datasets help models generalize better to unseen data.
- Support for Complex Tasks: Complex image classification tasks require detailed annotations, such as object boundaries, attributes, and contextual relationships.
Categories of Image Datasets
Image datasets for classification can be classified into several categories based on various criteria:
By Object Type
- Single Object Datasets: Contain images with a single object type, making them ideal for binary classification tasks.
- Multi-Object Datasets: Include images capturing multiple object types, providing a challenge for multi-class classification.
By Application Domain
- Medical Imaging: Datasets that focus on medical images for diagnosing diseases.
- Autonomous Vehicles: Datasets that include images of road signs, pedestrians, and vehicles.
- Social Media: Datasets sourced from user-generated content, encompassing various lifestyle images.
Creating High-Quality Image Datasets
Creating an effective image dataset for classification involves several crucial steps:
1. Define Objectives
Before gathering images, it’s vital to define what the dataset aims to achieve. Are you classifying objects, emotions, or scenes?
2. Collect Diverse Images
Use various sources to collect a diverse range of images to ensure that the model learns from a variety of perspectives and conditions.
3. Annotate Accurately
Engage skilled annotators or utilize advanced data annotation tools to label images accurately, maintaining consistency across the dataset.
4. Validate and Refine
Regular validation through sample checks ensures data quality. Refining the dataset by removing ambiguities enhances its effectiveness.
Keylabs.ai: Your Go-To Data Annotation Platform
At Keylabs.ai, we specialize in providing top-notch data annotation solutions tailored to your needs. Our platform offers an array of features designed to assist in creating high-quality image datasets for classification:
- Automated Annotation: Utilize our AI-driven tools for faster annotation without compromising quality.
- Customizable Workflows: Adapt our platform to fit your project's specific requirements.
- Team Collaboration: Facilitate seamless collaboration among team members, enhancing productivity.
With a commitment to quality and accuracy, we enable businesses to harness the full potential of data through precise annotation.
Best Practices for Using Image Datasets in Classification
To maximize the effectiveness of image datasets for classification, consider implementing the following best practices:
- Data Augmentation: Use techniques such as flipping, rotation, and scaling to artificially expand the dataset, improving model robustness.
- Regular Updates: Continuously update the dataset with new images to reflect changes and trends in the target domain.
- Performance Monitoring: Monitor model performance and analyze misclassifications to identify potential areas for dataset improvement.
Challenges in Working with Image Datasets
While working with image datasets, several challenges may arise:
Data Quality and Consistency
Maintaining a high level of quality across a large dataset is challenging. Discrepancies in labeling can lead to poor model performance.
Ethical Considerations
Using images from the internet raises questions of copyright and ethical use. Always ensure compliance with licensing requirements.
Computational Resources
Training models on large datasets requires significant computational resources. Investing in robust infrastructure is crucial for effective project execution.
The Future of Image Datasets for Classification
The future of image datasets for classification appears bright, driven by technological advancements and growing applications across various industries:
- Real-Time Annotation: Innovations in AI will likely lead to real-time annotation capabilities, reducing the time required for dataset preparation.
- Increased Use of Synthetic Data: The use of computer-generated images as training data will become more prevalent as methods for generating realistic imagery advance.
- Focus on Diversity and Inclusion: Future datasets will likely need to prioritize diverse representations to improve model fairness and reduce bias.
Conclusion
In the rapidly evolving landscape of artificial intelligence, image datasets for classification remain essential for developing accurate and robust machine learning models. By leveraging advanced data annotation tools and platforms like Keylabs.ai, businesses can enhance their data quality, improve model performance, and ultimately drive better outcomes. Embracing best practices and anticipating future trends will empower organizations to effectively navigate the challenges and opportunities presented by image data.