The Essential Role of Data Labeling in Machine Learning
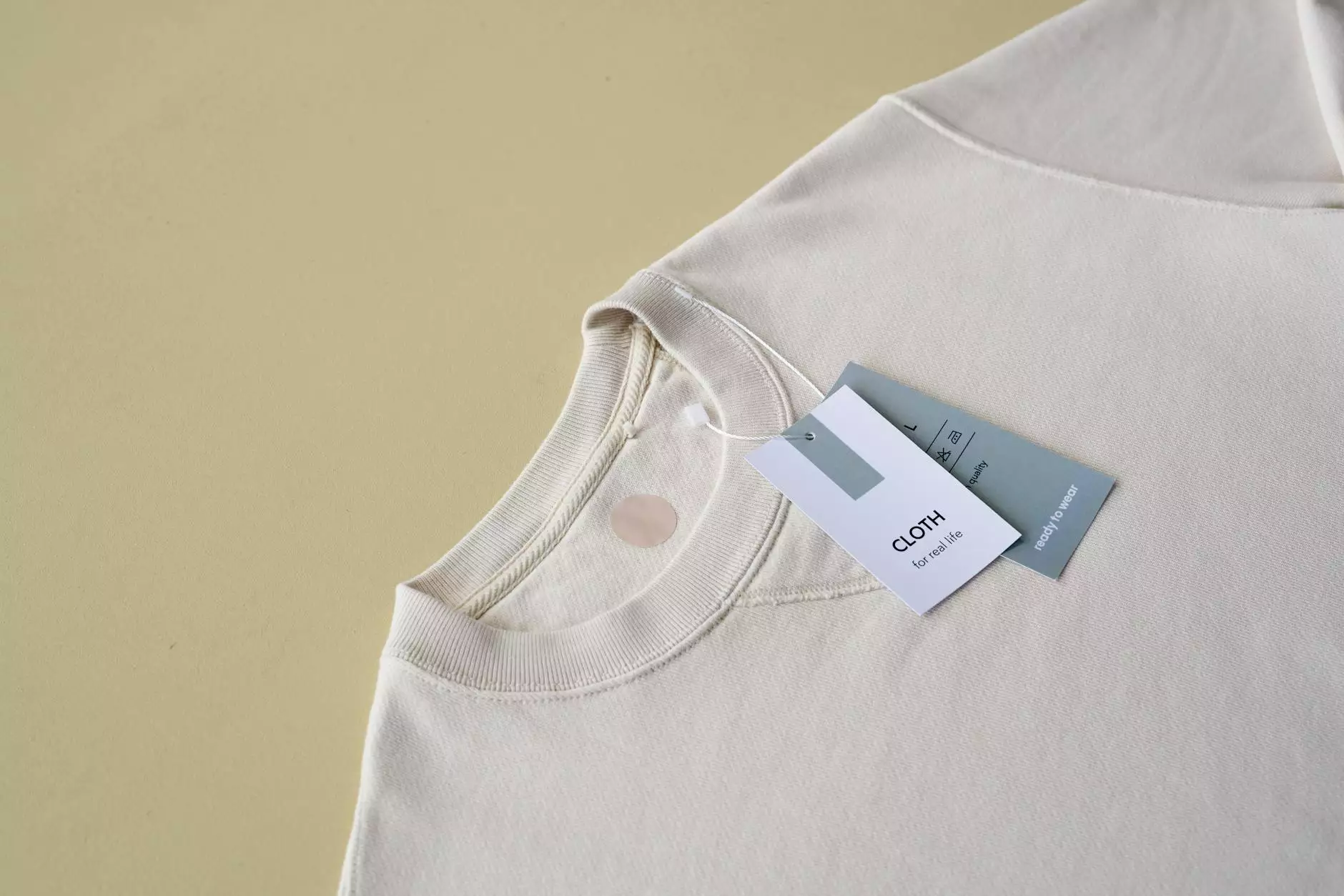
Data labeling plays a crucial role in the success of machine learning and artificial intelligence applications. It serves as the foundation upon which intelligent systems are built. As machine learning continues to evolve, understanding the significance of data labeling machine learning becomes increasingly important for businesses and developers alike. In this article, we will explore what data labeling is, its processes, the tools available, and the profound impact it has on various industries.
What is Data Labeling?
Data labeling is the process of annotating data to provide context and meaning. This involves assigning tags, labels, or categories to datasets, enabling machine learning algorithms to learn and make predictions based on that data. The significance of this process cannot be overstated in an era where data is growing exponentially.
The Importance of Data in Machine Learning
Machine learning algorithms rely heavily on large amounts of data to train and improve their accuracy. However, raw data alone is not sufficient. It must be structured and labeled appropriately to guide the learning process. The quality of labeled data directly influences the performance of machine learning models. Poorly annotated data can lead to inaccurate results and flawed decision-making.
Types of Data That Require Labeling
- Image Data: This includes labeling objects within images, segmentation tasks, and facial recognition.
- Text Data: Annotation of sentiment, entity recognition, and classification of various topics.
- Audio Data: This involves transcribing audio files and identifying speakers or environmental sounds.
- Video Data: Tracking objects in motion, activity recognition, and scene understanding.
The Data Labeling Process
The process of data labeling can be broken down into several stages:
1. Data Collection
The first step is to gather relevant datasets. This could involve scraping the web, using existing databases, or collecting raw data through various means.
2. Preprocessing
Before labeling, the data often needs to be preprocessed. This can include cleaning the data to remove noise, standardizing formats, or augmenting the data to expand the dataset size.
3. Annotation
This is where the actual labeling occurs, and it can be performed manually or automatically. Manual annotation involves human annotators reviewing and labeling data, while automated tools use predefined rules and algorithms to label data.
4. Quality Assurance
Quality assurance is vital to ensure the accuracy of labels. This can involve cross-validation, where multiple annotators label the same data, or utilizing software to check for consistency.
5. Integration into Machine Learning Models
Finally, the labeled data is integrated into machine learning models for training. This stage is critical as it determines how effectively the models will learn from the data.
Data Annotation Tools and Platforms
With the growing demand for high-quality labeled data, numerous data annotation tools and platforms have emerged to facilitate the process. Keylabs.ai stands out among these platforms for several reasons:
1. User-Friendly Interface
Keylabs.ai offers an intuitive interface that simplifies the data labeling process. Users can quickly navigate through the platform, making it easy to annotate data without extensive training.
2. Quality Control Features
The platform includes built-in quality control features, ensuring that every label meets a high standard. This reduces the likelihood of errors and enhances the reliability of the data.
3. Scalability
As projects grow, so does the need for larger datasets. Keylabs.ai provides scalable solutions that can accommodate projects of any size, making it suitable for startups and enterprise-level applications alike.
4. Support for Various Data Types
Whether you're working with images, text, audio, or video, Keylabs.ai supports a range of data types, enabling businesses to label data that is most relevant to their needs.
The Future of Data Labeling in Machine Learning
The role of data labeling in machine learning is continually evolving. With advancements in technology, we can expect the following trends:
1. Increased Use of Automation
As machine learning techniques advance, automated data labeling tools are becoming more sophisticated. These tools can drastically reduce the time and cost associated with manual labeling while maintaining high accuracy rates.
2. Integration of AI in Labeling
Artificial intelligence is expected to play a more significant role in data labeling, assisting human annotators and even taking on complex labeling tasks independently.
3. Emphasis on Ethical AI
As AI systems become more integrated into daily life, the need for ethically sourced and labeled data will rise. Companies will need to ensure that the data they use does not perpetuate biases or ethical concerns.
4. Expanding Applications Across Industries
The use of labeled data is not limited to tech companies. Industries such as healthcare, finance, and retail are increasingly leveraging data labeling to improve their operations, personalize customer experiences, and enhance decision-making.
Conclusion
In conclusion, the significance of data labeling machine learning cannot be overstated. It is the backbone of successful machine learning projects, ensuring that algorithms learn from accurately annotated data. Businesses that invest in data labeling tools and platforms, such as those offered by Keylabs.ai, are poised to leverage the power of AI effectively.
As technology advances and the amount of data continues to grow, staying ahead in data labeling will be crucial. Organizations must embrace the best practices and tools available to harness the full potential of machine learning and artificial intelligence, paving the way for smarter and more efficient business solutions.