Label Images for Object Detection: The Ultimate Guide
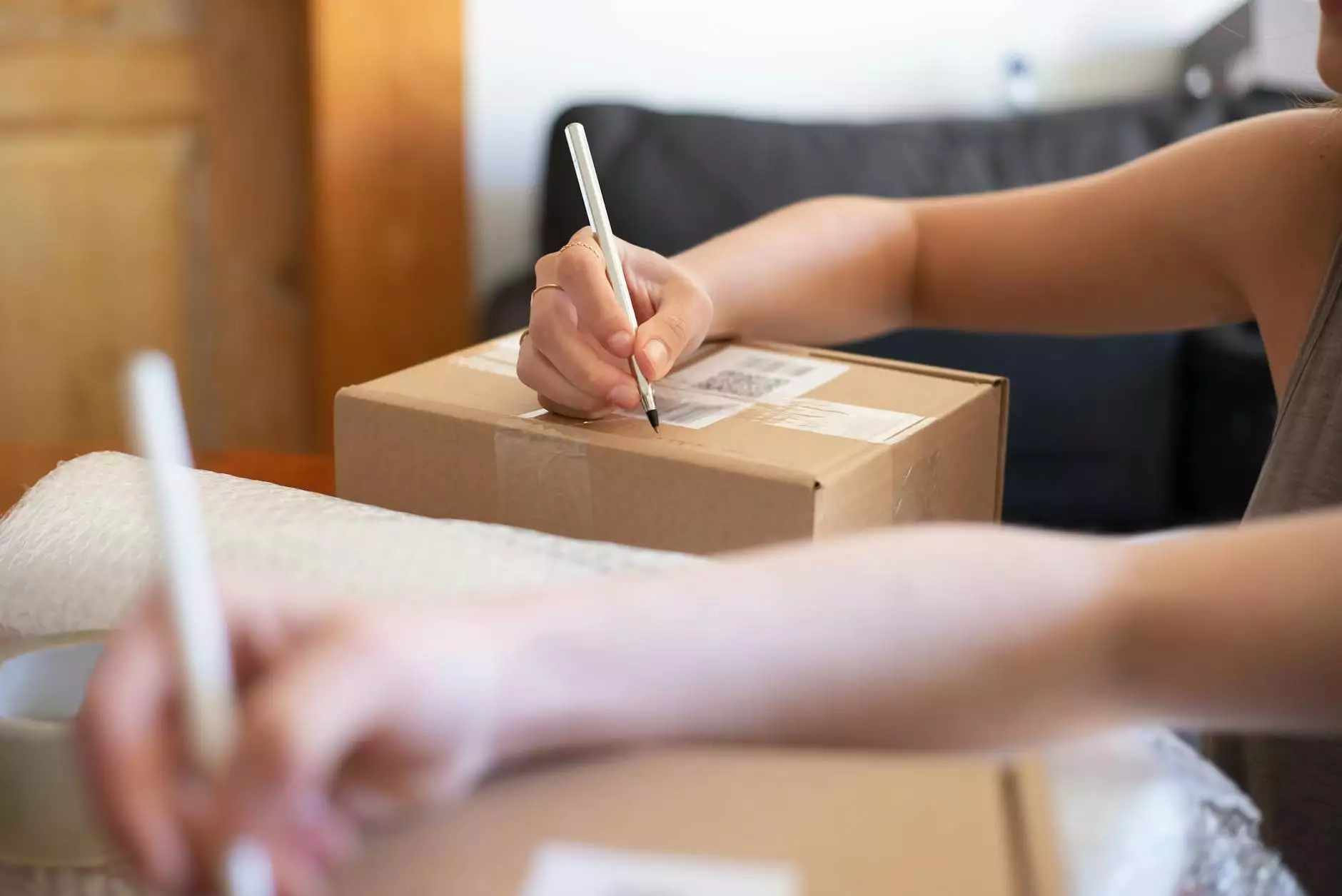
In the rapidly evolving field of artificial intelligence and machine learning, the ability to label images for object detection has become a critical component. As organizations seek to leverage cutting-edge technologies, ensuring data is accurately annotated has emerged as a required skill. This comprehensive article from Keylabs.AI seeks to explore the aspects of data annotation, best practices, and how our tools can facilitate high-quality output.
Understanding Object Detection
Object detection is a sub-field of computer vision that involves identifying and locating objects within images and videos. It combines both classification and localization tasks. For machines to discern various objects, they must learn from accurately labeled datasets. Thus, effective labeling serves as the bedrock of successful object detection models.
The Importance of Labeling
Labeling images forms the foundation for supervised learning, where models learn patterns based on provided datasets. Proper labeling ensures:
- Increased Accuracy: Accurate labels yield more precise model predictions.
- Improved Training Efficiency: Well-annotated datasets accelerate the training process.
- Better Model Interpretability: Clear annotations allow for easier model evaluation and understanding of decision-making processes.
Best Practices for Labeling Images for Object Detection
To successfully label images for object detection, it is essential to follow industry best practices. Below are several guidelines to ensure high-quality annotations:
1. Utilize the Right Annotation Tools
Selecting the right data annotation tool significantly impacts the labeling process. At Keylabs.AI, we offer state-of-the-art platforms designed to streamline the annotation process. These tools provide intuitive interfaces that enhance productivity and ensure accuracy.
2. Define Clear Labeling Guidelines
Establish clear guidelines detailing how objects should be labeled. This includes:
- Determining the level of granularity.
- Specifying how to handle occlusions and overlapping objects.
- Standardizing labels across different jobs to maintain consistency.
3. Ensure Quality Control
Implement quality control measures to review annotations periodically. Utilizing a feedback loop where annotators can learn from mistakes can significantly improve output quality over time. This strategy enhances the overall reliability of the dataset.
4. Leverage Automation Wisely
While manual annotations are often necessary, leveraging automated tools can help speed up the process. Nonetheless, it is vital to review the output of automated labeling to ensure it meets quality standards.
Labeling Techniques for Object Detection
There are several techniques for labeling images effectively, especially when preparing datasets for object detection.
Bounding Box Annotation
This technique involves drawing a rectangle around the object of interest. Bounding boxes are essential for detecting localized objects within a scene.
- Advantages: Simple to implement and effective for many object detection tasks.
- Best Use Case: Situations where objects have clear edges and are distinguishable.
Polygon Annotation
For irregularly shaped objects, polygon annotations offer a more fitting approach. This method allows for a complex outline that tightly wraps around an object.
- Advantages: More precise and provides comprehensive object representation than bounding boxes.
- Best Use Case: Articulated objects, such as vehicles, animals, or any objects that do not conform to rectangular shapes.
Semantic Segmentation
This labeling technique involves assigning a label to every pixel in the image. It offers the most granularity in classification, as it details the exact position and shape of every object.
- Advantages: High precision and full object outlines.
- Best Use Case: Detailed object recognition tasks where the distinction between objects is crucial.
Why Choose Keylabs.AI for Data Annotation
At Keylabs.AI, our mission is to empower businesses with advanced data annotation solutions that drive machine learning success. Here are just some reasons why we are a top choice:
Advanced Tools
Our data annotation platform is equipped with cutting-edge features designed to simplify the annotation process and increase efficiency. From intelligent tagging to collaborative workspaces, our tools are top-notch.
Expert Team
We have a dedicated team of skilled annotators who understand the nuances of labeling for object detection. Their expertise ensures your data is annotated with the highest standards of quality.
Custom Solutions
No two projects are the same. Our team collaborates with clients to create customized solutions tailored to specific needs, ensuring you receive the best possible support.
Conclusion
In conclusion, labeling images for object detection is not just a simple task; it’s a critical process that lays the groundwork for the accuracy and effectiveness of machine learning models. By employing the right tools, following best practices, and choosing an experienced partner like Keylabs.AI, your data annotation efforts will yield significant results and provide valuable insights for your organization. Embrace the power of accurate data labeling and transform your image datasets for effective object detection today.